Do Analytics Say that Andrei Vasilevskiy is Overrated?
Does the Vézina-winning goalie deserve the scorn he gets from some analytically-minded fans?
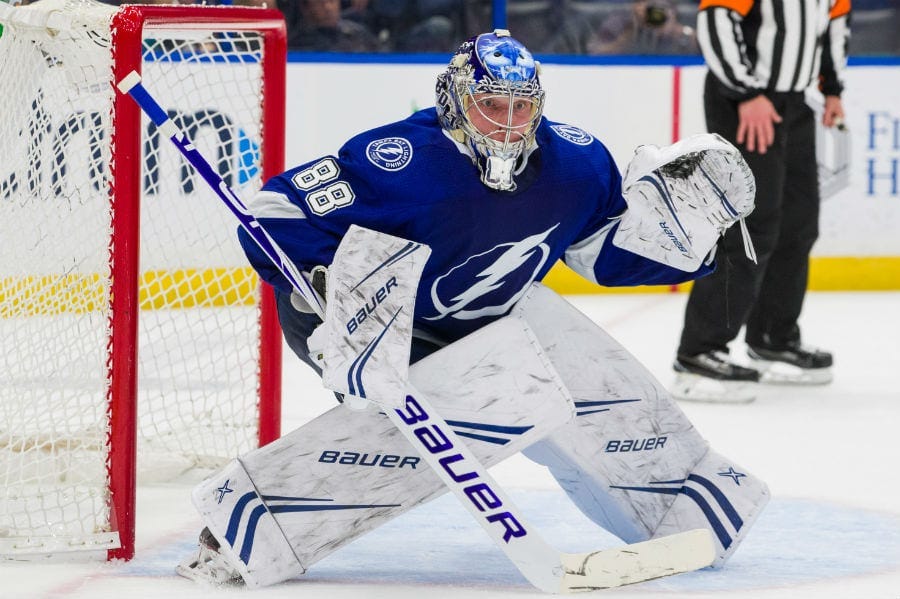
Sometimes a take comes around that’s just so appealing that you can’t help but hop on board and shout it from the rooftops - especially when there seems to be solid evidence behind it. I think that “Andrei Vasilevskiy is actually bad” is a perfect example: the idea that a guy with a Vézina Trophy making $9.5M per season playing on the league’s most dominant team is a total fraud is a fun contrarian opinion to have; I certainly enjoyed having it. Many people who use analytics to guide their evaluation of players (and goaltenders in particular) have adopted this view based on Vasilevskiy’s relatively poor “versus expectation” stats as listed on EvolvingHockey.com (in my mind, the best hockey analytics site out there), including me up until recently. But I’m starting to think it’s a little bit more complex than that.
To properly analyze a goalie you have to use stats that isolate them from the defence in front of them as much as possible (because it’s not Connor Hellebuyck’s fault if Kevin Chevaldayoff forgot to acquire anybody who can actually defend last summer). The best way to do this is to use “above expected” stats which compare what goals a goalie did allow to what a goalie would be expected to allow based on the quality and quantity of the shots he faced. You can do this with a rate stat (save percentage above expected), or if you want to reward goalies who have played more, you can use a cumulative stat (goals saved above expected). In principle, this is the best and, in my opinion, only way to properly evaluate a goalie - where complications arise is actually calculating it.
Many people who are a lot smarter than I am have built models designed to measure goaltending based on this principle. Some of them are proprietary, meaning we don’t have the ability to scrutinize their methodology or most of their results. The three most prominent public ones come from EvolvingWild (who you’d recognize from creating WAR and the RAPM isolates I often use), MoneyPuck, and CrowdScout. Because they are built to account for different things, they occasionally have differing opinions on goalies and rankings. I won’t go into too much detail on the subtle differences between them, but there are two key ones that are relevant to Vasilevskiy: arena bias and rebounds.
Arena Bias is based on the observed differences between how different arena trackers measure shot distance. Because the NHL tracks stats manually (at least until they get around to finally actually getting those microchips into pucks), there’s room for human error in the measurements. For example, in the early 2010s there was a massive difference between the average shot distance that Rangers goalies faced at home compared to on the road. This led to Henrik Lundqvist recording wildly inflated GSAx numbers, because expected goal models trusted the NHL’s shot data and overstated how difficult the shots he faced were. While this isn’t as much of a problem now, there are still a handful of stadiums that have issues with this. MoneyPuck and CrowdScout adjust for this, but EvolvingWild do not.
Rebound Adjustments are based on the principle that a goalie shouldn't be statistically rewarded for allowing and then saving avoidable rebounds. A goalie with strong rebound control might actually end up with worse GSAx than an otherwise identical one who allows plenty of them, because by allowing extra opportunities he is creating more chances against. MP features a “flurry adjustment" which identifies when a whole whack of shots and rebounds take place in quick succession, while CrowdScout go all the way in filtering out “rebounds above expected.” EvolvingWild’s does not. Some people have qualms with the concept of rebound adjustments, and it’s probably the most “controversial” element of GSAx modelling. You can read Cole Anderson’s full explanation for why he uses such an adjustment here.
Both of these affect Vasilevskiy, and lead to a vast difference between his standing in EW's model compared to the other two. Firstly, there's good reason to believe that Tampa does the opposite of what MSG used to do, underestimating the distance of shots that the Lightning take and face (in the past three years, they rank 3rd and 2nd in difference between home and away xSv% and xSh% respectively.) That means that the expected goal value of the shots Vasi faces is less than it should be, which hurts his GSAx when he does allow goals.
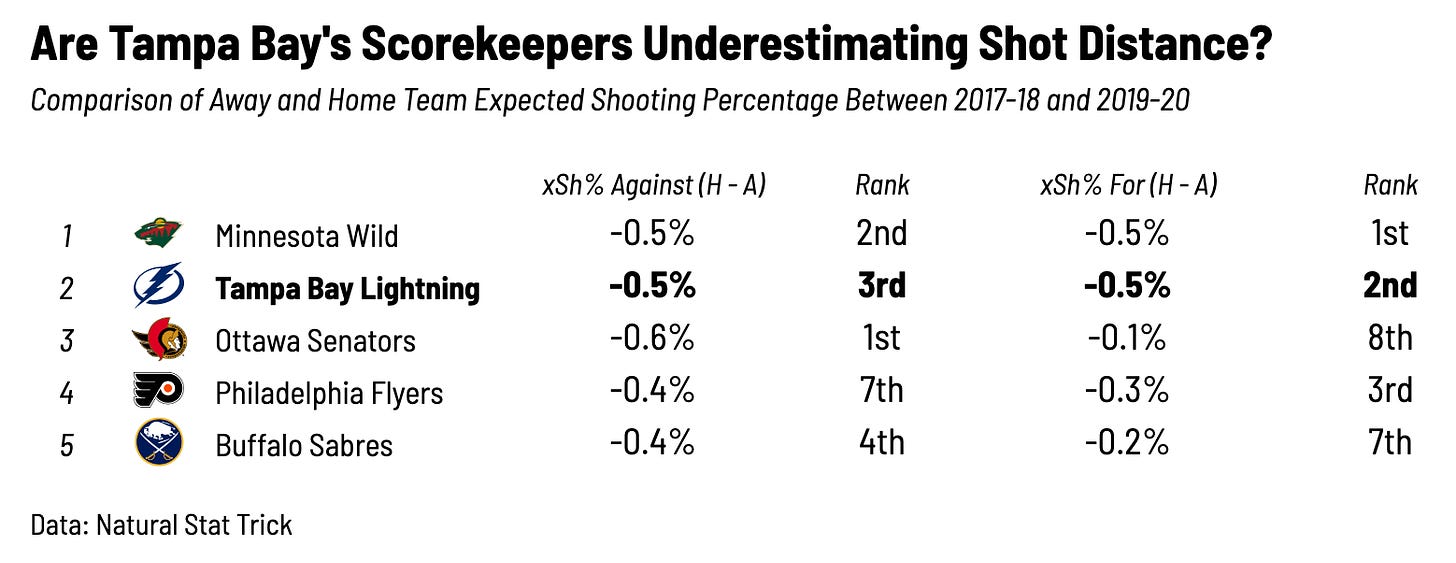
Secondly, Vasilevskiy exerted very strong rebound control this season, ranking 5th among starters in MoneyPuck’s model and 3rd in CrowdScout’s at preventing rebounds above expected. Adjustments of this degree are not consensus in the analytics community by any means, and it remains impossible to truly measure the “preventability” of a rebound. However, given the principle of goaltending analytics (to strip away all elements of the game outside of the player’s direct control) it does make intuitive sense not to credit goalies with saves they made only because of their own error. On top of that, rebound-adjusted dSv% figures have been found to be slightly more predictive than unadjusted ones (both by Cole Anderson himself and by my own rudimentary tests)
As a result, check out the dramatic differences between how he ranks among the league’s 31 starting goalies in dSv% (Save % Above Expected) across the three models.
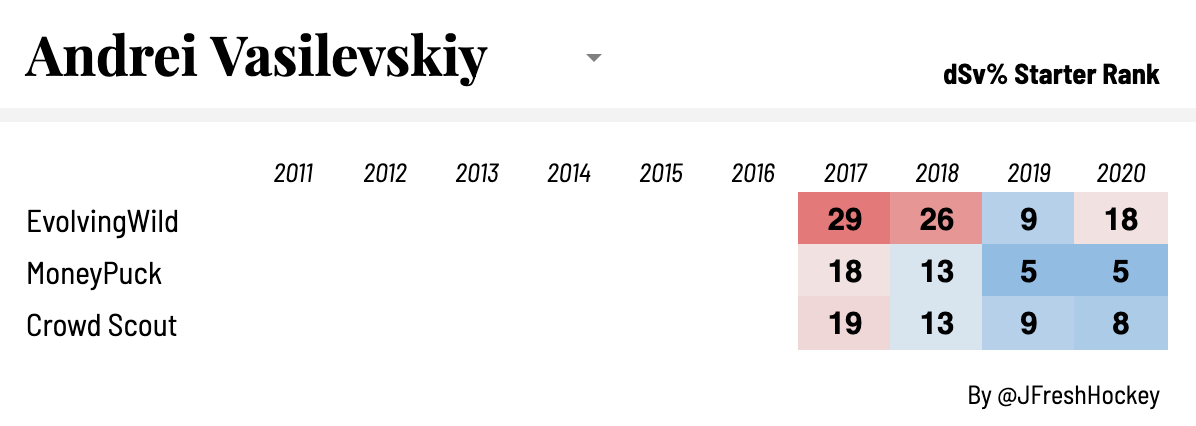
With the exception of last season, one model is a clear outlier. So I think there should be a little more hesitation when it comes to denouncing Vasilevskiy as yet another wins-over-everything fraud who’s riding his team to an underserved reputation. Is he the best in the league, or even an elite goalie? Probably not. Was it smart for the Lightning to lock up a goalie to a longterm $9.5M a year contract? Almost definitely not. But he’s also probably not a well-below-average starter like some (including me two months ago) would have you believe.
The only overrated person here is you