How Should We Interpret Microstats?
Manually tracked data is incredibly useful, but be careful when drawing conclusions from it.
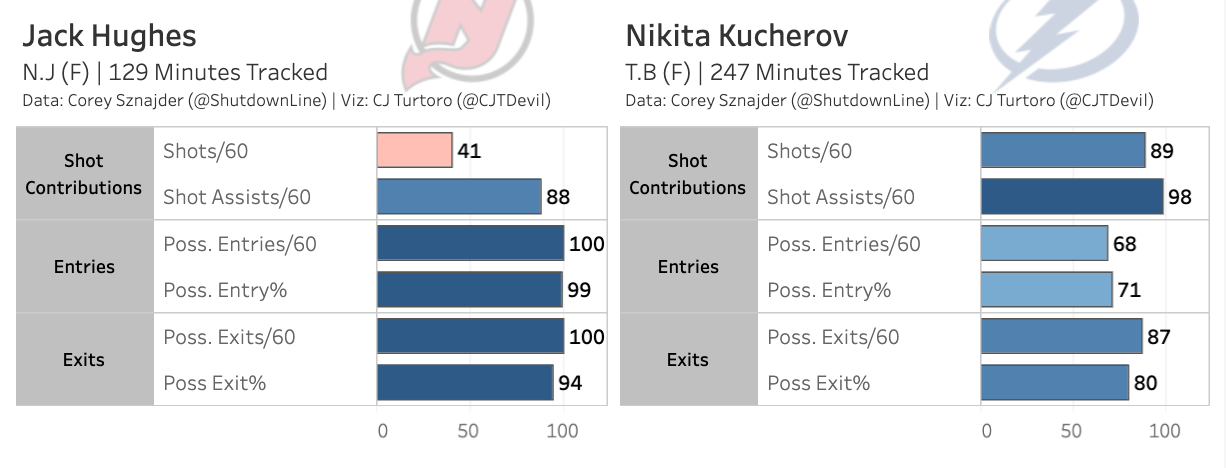
Among the large and growing body of publicly available hockey analytics, one of the most impressive sources is Corey Sznajder’s All Three Zones project. By watching games and “tracking” them - that is, manually recording almost everything that happens into a spreadsheet - Corey has built a resource that has powered numerous studies of how the game works and countless evaluations of players and teams. His exhaustive efforts to provide detailed and organized microstats to those lacking the resources of an NHL franchise has resulted in a database including all of the stats listed below for the past four seasons as well as the playoffs for hundreds of players:
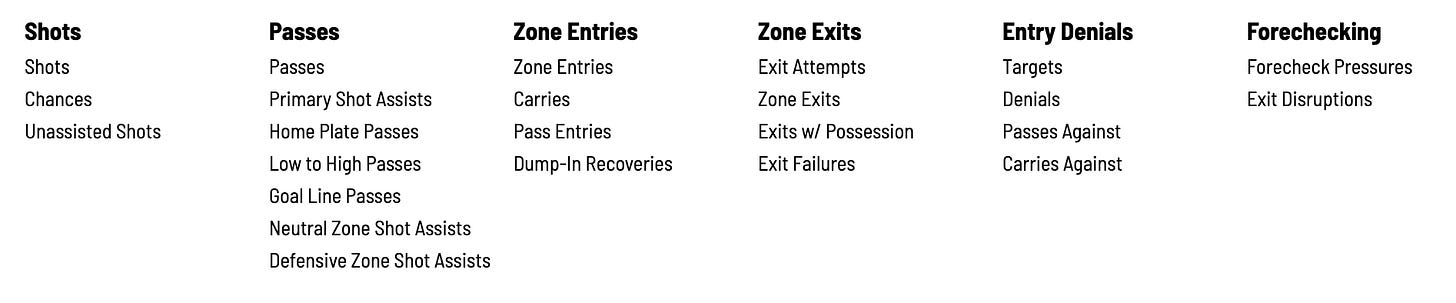
Without his work, we would be essentially be flying blind when it comes to understanding how players and teams play and how they achieve the results that they do. For example, without access to forechecking and zone entry data, figuring out why Zach Aston-Reese and Valeri Nichushkin had such insane defensive impacts would have been much trickier. By turning the imprecision of the “eye test” into something concrete and quantitative, we can compare players to their peers in categories that would otherwise be nebulous; sure, it’s clear from watching Hurricanes games that Jaccob Slavin breaks up a lot of zone entries, but how does he compare to Josh Morrissey in that regard? Having microstats mean you don’t have to talk out of your ass. CJ Turturo has helped make this data accessible to even more fans by visualizing Corey’s data on Tableau, and his A3Z Dashboard has become one of the most-cited visualizations out there.
At the same time, however, when multiple sources of data exist there will be always be the possibility of misinterpretation and even cherrypicking. This can especially be the case when the stats in question are new to people. As somebody who writes about players all the time, figuring out how best to interpret these numbers has been a difficult but necessary task to make sure I’m not misrepresenting what they actually mean. With free agency around the corner, I thought it would be a good time to address some errors I’ve noticed myself and others make when using them. These principles could also be applied to the interpretation of privately tracked microstats, but as they’re only occasionally presented to us I decided to focus on more accessible ones.
Microstats Measure Events, Not Outcomes
The main mistake I often see people make when using the A3Z visualizations is using them to evaluate how good a player is overall. In part, I think this is because our brains have been trained to look at hockey-related bar graphs and associate blue bars = good, red bars = bad, ergo a player with lots of blue bars is good. But because microstats serve a specific function, this isn’t really the case.
Let’s use Tyson Barrie as an example. When the Leafs traded for Barrie in summer 2019, the combination of his reputation and Dubas’ analytical acumen led to quite a bit of suprise when the outputs of Micah McCurdy’s and EvolvingWild’s macro-level models were less than favourable to the defenceman. Here’s Barrie’s 2018-19 RAPM chart, per EvolvingHockey.com:
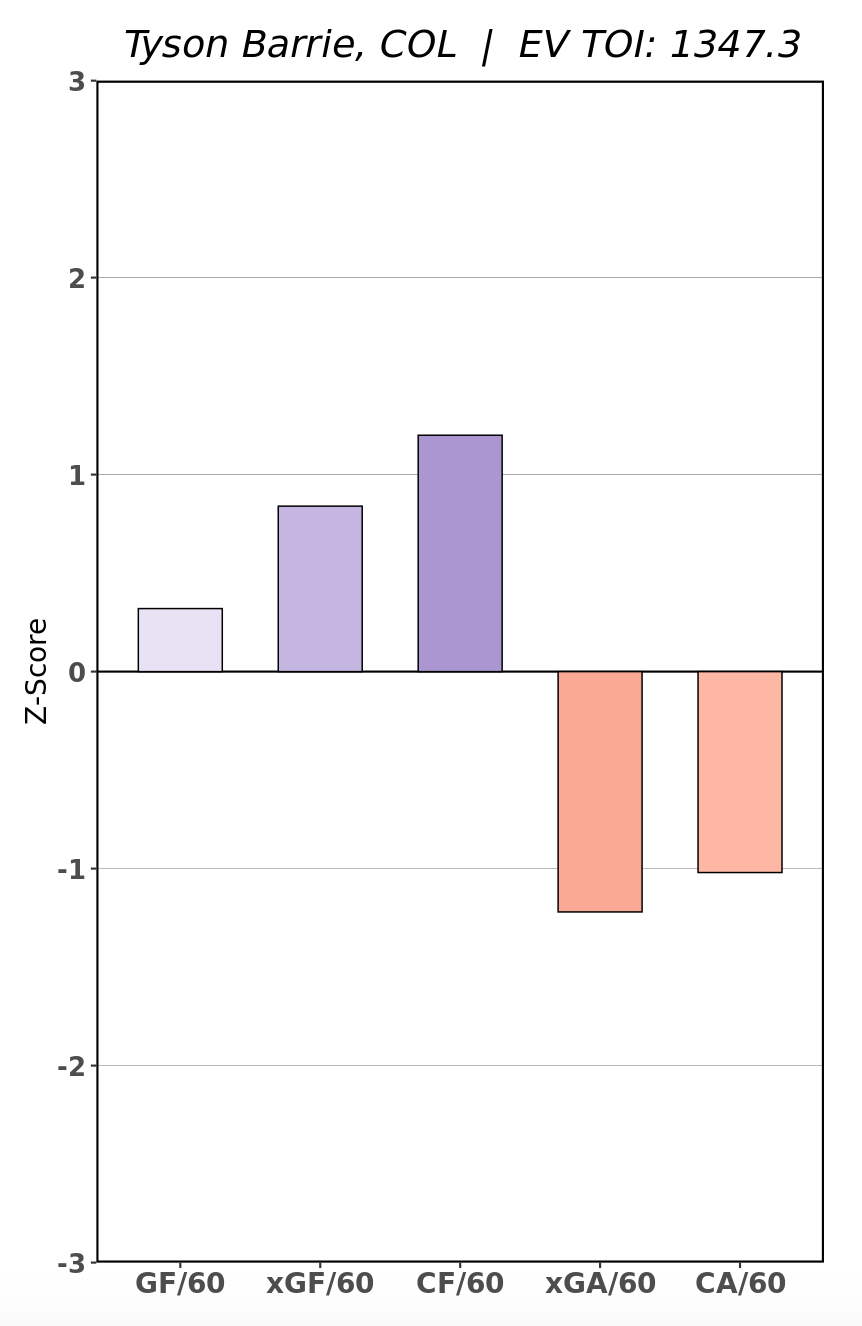
This would suggest that Barrie’s defensive play is so bad that it cancels out his offensive ability and renders him a below-average defenceman. If you’re a Toronto Maple Leafs fan, this isn’t what you want to hear. But then you see this chart:
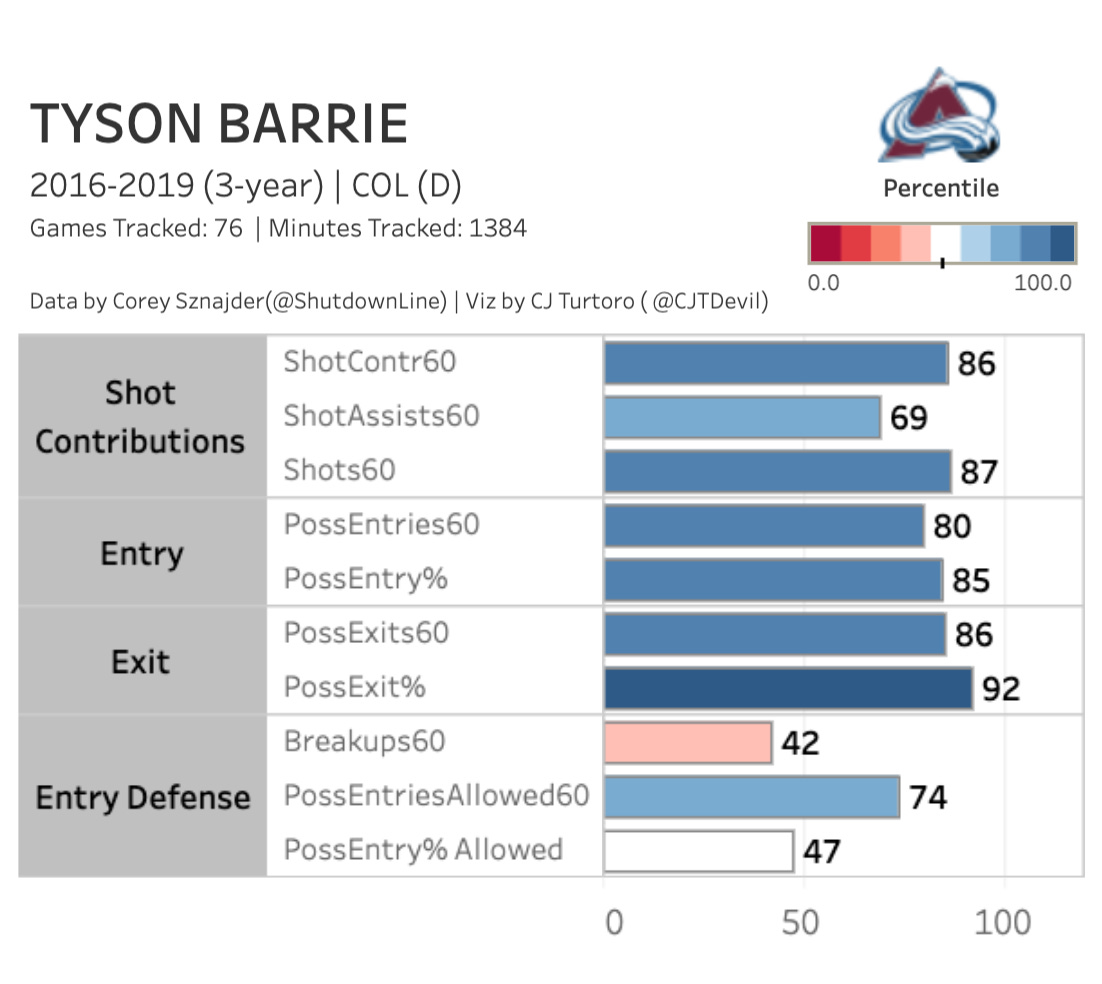
Look at all the blue! Look at all the 80s and 90s! A very real tendency exists to use data to serve whatever pre-existing opinion you have - a.k.a. confirmation bias. If you have two colourful analytical graphs telling you seemingly opposite things (Barrie is mediocre vs. Barrie is excellent), you’re probably going to defer to the one that says what you want to hear.
But these two charts don’t contradict eachother. They combine to tell a more complete story about Barrie’s results, not a conflicting one. The latter visualization shows that Barrie is an active player in transition, entering and exiting the neutral zone with possession of the puck frequently and at a high rate. It also says that he takes a lot of shots and passes the puck a decent amount in the offensive zone. This might be one of the reasons that his offensive performance is above-average, as measured by the former chart. What’s missing is the bulk of what happens in the offensive zone and any of what happens in the defensive zone - the primary weakness of Barrie’s game. And yet I remember seeing many instances of Leafs fans deferring to the A3Z chart in defence of the Barrie acquisition rather than combining the two to better understand the type of player their team had traded for. And sure enough, he turned out to be a slightly worse version of the player that the RAPM chart suggested he would be, and sure enough, Leafs fans were very unhappy with that.
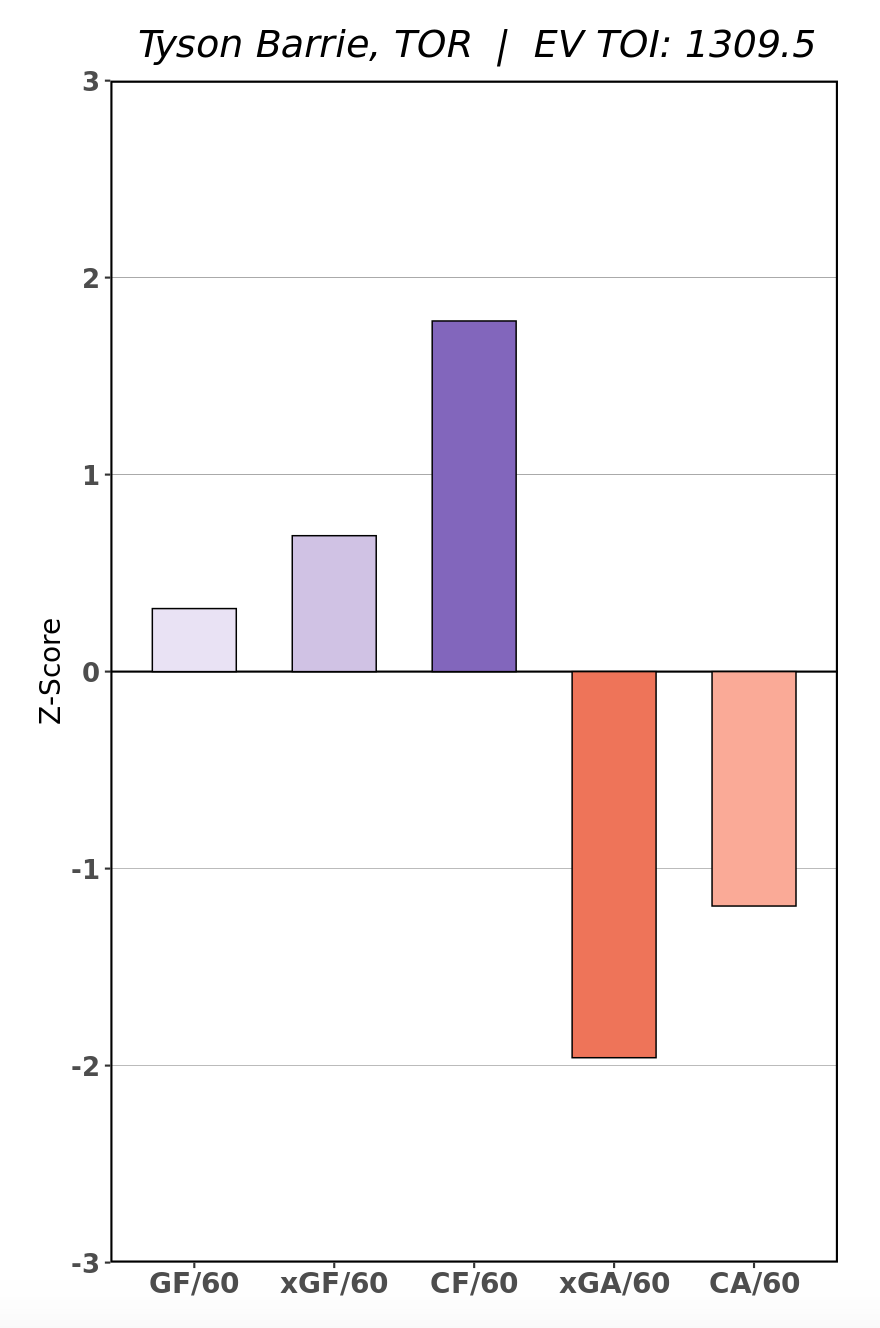
For an even more recent example, Penguins acquisition Mike Matheson is not a player that macro-level models are particularly fond of, but you wouldn’t guess it from his A3Z chart:
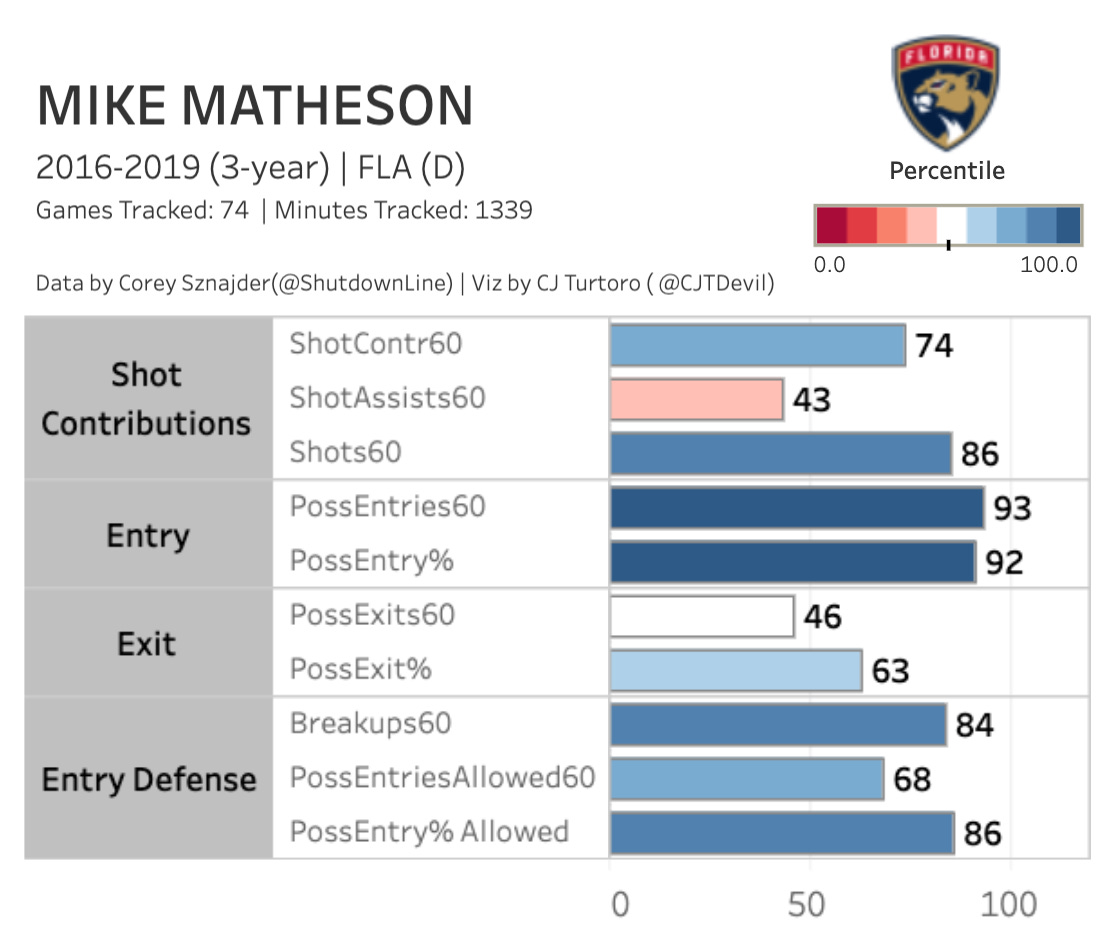
I saw a lot of analytically-inclined Pittsburgh fans cite this viz as evidence that he’s a much better player than his impacts suggest and could rescue the team’s transition game. But look more carefully and you can see that his biggest strength is entering the offensive zone with possession of the puck. As the clip below demonstrates, leading the charge as a defenceman isn’t necessarily a desirable attribute, especially if the player in question lacks the playmaking ability and puck control to generate anything once inside the zone:
So to summarize, don’t just defer to whatever chart is more encouraging and don’t mistake events for outcomes. Remember that the objective in hockey is to score more goals than your opponent, not accumulate the most zone entries with possession. Some excellent transition players are useless in their own end and the offensive zone. Some of the best defensive defencemen in the NHL give up the blueline easily. Microstats describe the road, not the destination.
Microstats Measure Events, Not Ability
It can be equally tempting to use microstats to declare somebody’s talent in certain areas. They would appear to allow us to do things like rank the best transition players or pick the top ten best defencemen at defending their blueline. You could almost think of it like NHL 20 attributes: Player X has a 95 puck control rating because he ranked third in controlled zone entries.
But this ignores how much coaching and systems influence these events. Players don’t make decisions based purely on an evaluation of their own ability to perform certain tasks, nor do they all value the same thing. Possession entries might be statistically more likely to create a shot, but that doesn’t mean crossing the blueline with the puck is always the best option - the Lightning were a below-average team in that regard but assembled a group of tenacious puck retrievers that made a forechecking-based game effective. Challenging opponents at the blueline can be extremely risky, and some great defensive teams like the Islanders and Blue Jackets prefer their defencemen to play more conservatively and keep opponents to the perimeter on the rush.
As an example, take a look at Shayne Gostisbehere’s A3Z charts in 2018-19 and 2019-20:
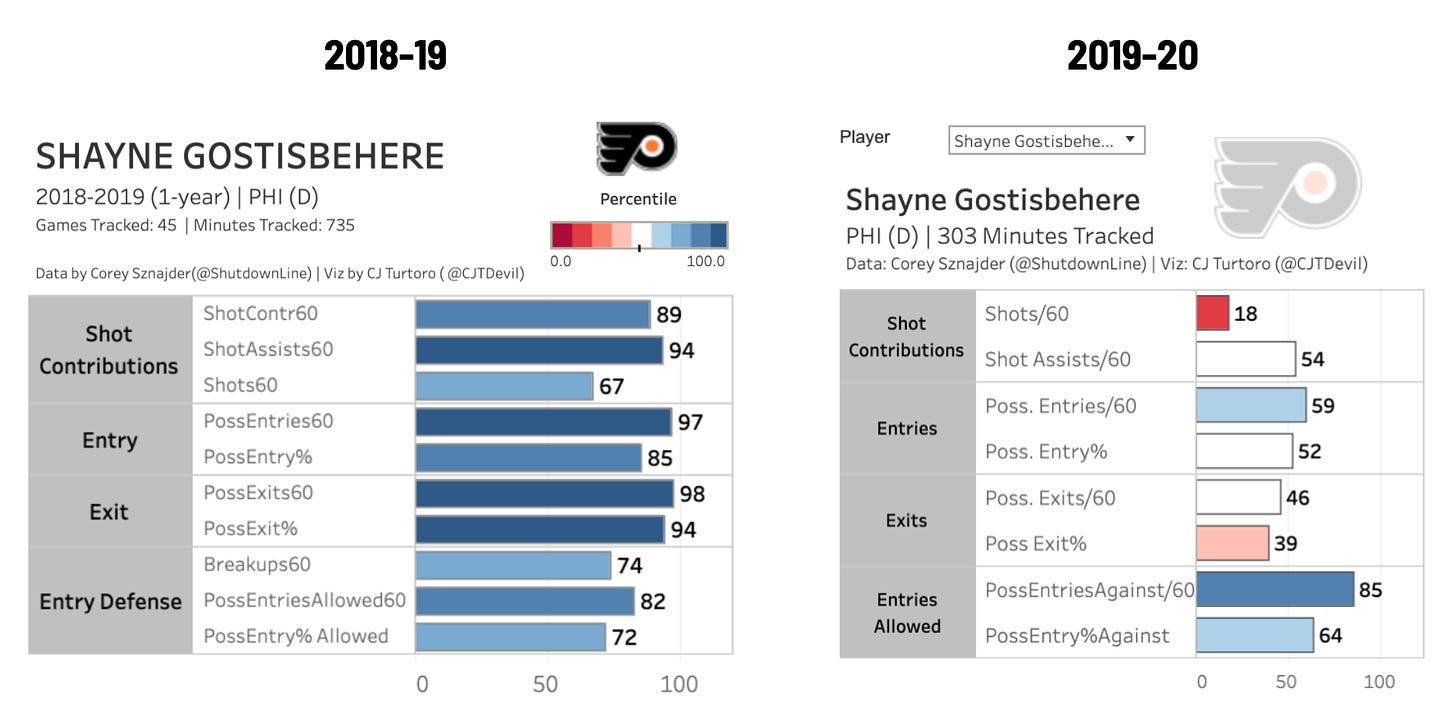
Did Shayne Gostisbehere forget how to make a breakout pass over summer vacation in 2019? That seems pretty unlikely to me. But if you were making ability judgements based on these charts, that would be the logical conclusion.
What actually happened is that the Flyers hired a new coach, Alain Vigneault, who brought with him a new, more conservative system. Per Sznajder’s data, they went from a team that valued maintaining control of the puck in transition to a below-average possession exit squad and one of the most dump-and-chase-heavy teams in the NHL. A guy like Gostisbehere didn’t really fit, and accordingly finds himself on the trade block as a result.
Like any hockey analytic, players’ microstat numbers describe what happened. They do not objectively measure ability in any meaningful sense. We can interpret them to analyze what certain players are capable of, whether they’d be a fit on a certain team, etc., but be skeptical when somebody tells you that a certain player is a poor puck carrier or weak on the blueline based purely on their tracked stats. One thing that I’ve found extremely useful is to combine the A3Z charts with Sznajder’s own visualizations, which show the entire league and teams at once instead of individual players. This lets you see how players function as part of their team’s system rather than independently.
Conclusion
Few resources match Corey Sznajder’s tracked stats in terms of their value to fans trying to gain a better understanding of how players and teams play the game. We are very fortunate to have access to this data, which requires hundreds of hours of work to compile and reveals information that would otherwise be restricted to teams and broadcasters. Microstats can deepen our analysis but like any stat, if interpreted incorrectly or in bad faith, they can also mislead. Only recognizing them for what they are and what their value is, rather than taking them as measures of a player’s effectiveness or ability, will ensure that they are used to their full potential.